The Applied Intelligence Research Centre has a diverse team.
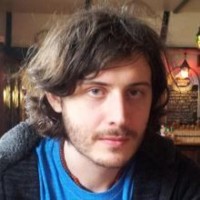
A generalization bound is any theoretical result guaranteeing that with high probability, a given supervised learning system will produce at least a certain test accuracy when given a certain number of samples. In other words, it is a confidence bound for the system's test error. It is well-known (see e.g. Zhang et al. 2017) that classical bounds from statistical learning theory are vacuous for neural networks, this is essentially because these bounds are in a certain sense worst-case, and neural networks typically operate far from that worst-case in the settings where they are applied. I am interested in understanding modern approaches to the generalization bound problem for neural networks, understanding whether these approaches can be improved or if they are inherently limited in similar ways to classical bounds, and exploring which approaches work best in specific settings (architecture, dataset).